Is ChatGPT bias? Ask OpenAI.
- Aalya Dhawan
- Jan 12, 2023
- 4 min read
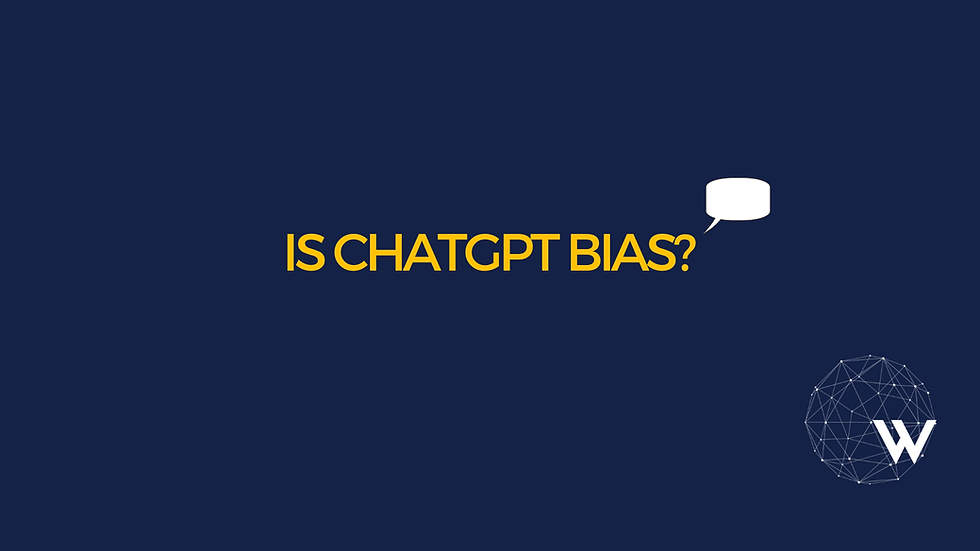
The buzz is evergrowing with this new tool taking the world by storm. AI bias is an open truth and we wanted to see if the new technology understands the predicament of this bias in the realm of Artificial Intelligence. See the results for yourself.
Prompt: Is ChatGPT bias?
Bias in machine learning models, including language models like ChatGPT, has been a topic of much discussion in recent years. Bias in these models can lead to incorrect or unfair predictions, which can have serious consequences in areas such as employment, lending, and healthcare. In this essay, we will explore the ways in which bias can be introduced into language models like ChatGPT, the potential consequences of bias, and the efforts being made to address it.
First, it is important to understand how bias can be introduced into a language model like ChatGPT. The model is trained on a large dataset of text, known as the training data. This data is used to teach the model the patterns and relationships between words and phrases. However, if the training data contains biases, the model will learn and replicate those biases in its responses. For example, if the training data contains a disproportionate number of examples of a certain gender or race, the model may perpetuate stereotypes or make biased assumptions about those groups.
One of the main sources of bias in language models is the training data itself. The data used to train ChatGPT and other models is often sourced from the internet, which can contain a wide range of views and opinions. This can include biases and stereotypes that are present in society. Additionally, the data used to train the model may not be representative of the population that the model will be used for. For example, if a model is trained on data from the United States, it may not perform as well when used in other countries.
Another source of bias in language models is the way the model is designed and trained. For example, some models may be designed to make certain types of predictions or to prioritize certain types of information. This can lead to biases in the model's responses. Additionally, the way the model is trained can also introduce bias. For example, if the model is trained on data that is labeled by humans, the labels may reflect the biases of the labelers.
The consequences of bias in language models like ChatGPT can be significant. For example, a biased model may make incorrect predictions about a person's qualifications or abilities, leading to unfair hiring decisions or lending practices. In healthcare, a biased model may make incorrect diagnoses or treatment recommendations, which can have serious consequences for patients. Additionally, bias in language models can perpetuate and amplify existing societal biases and discrimination.
To address the issue of bias in language models like ChatGPT, researchers and developers are taking a number of steps. One approach is to use more diverse and representative training data. This can help to reduce the impact of biases that may be present in the data. Additionally, researchers are working on techniques to detect and remove biases from the training data. For example, researchers are using techniques such as adversarial training, which helps the model to learn from a wider range of examples, and debiasing methods, which helps the model to correct for biases in the training data.
Another approach is to use techniques that help the model to be more robust to bias. For example, researchers are developing methods for the model to be more aware of the context in which it is making a prediction. This can help the model to understand when a bias may be present and to make a more accurate prediction. Additionally, researchers are developing techniques for the model to be more transparent, so that users can understand how the model is making a prediction and identify potential biases.
In addition to these technical solutions, there is a growing awareness of the need for more diverse and inclusive teams in the development and deployment of such models. A diverse team with different backgrounds and perspectives is more likely to identify and address potential biases in the model.
In conclusion, while ChatGPT is not inherently biased, the data it was trained on may contain biases which could be reflected in the model's responses. However, researchers and developers are actively working to identify and mitigate these biases in language models. This includes using more diverse and representative training data, developing techniques for detecting and removing biases from the training data, and designing models that are more robust to bias. While there is still much work to be done, these efforts will help to ensure that language models like ChatGPT are as unbiased as possible and continue to provide valuable and accurate information to users.
So, what do you think? Is it bias? Comment your thoughts. Let us know what kind of content you'd like to see! We would love to create.
Follow us for more such content and join our community.
I haven't found any clear evidence of bias yet, but with such a powerful tool , in a research preview phase, it could take a while to find it! Very proper response to my question "Are boys better at maths than girls?" "There is no evidence to suggest that boys are inherently better at math than girls. Research has shown that girls perform just as well as boys in math when given the same opportunities and resources. Studies have also shown that girls who are encouraged and supported in their math studies perform just as well as boys. There are also many successful women in math-related fields, such as engineering and physics.
However, it is true that there is a…